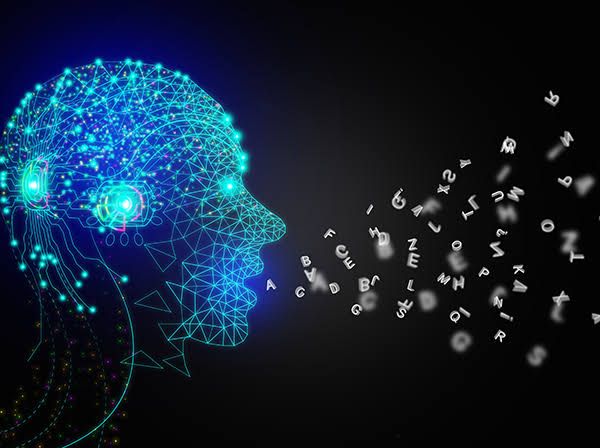
Generative AI systems are rapidly transforming the way we create content, from generating articles and scripting videos to crafting social media posts and even artwork. Technologies like OpenAI’s GPT models, Google’s Bard, and other generative AI tools are increasingly being integrated into various industries for their efficiency and scalability. However, as we lean more on these tools, ensuring the accuracy and ethicality of their output has become a pressing concern.
This blog dives into why controlling the output of generative AI matters and explores challenges, solutions, and best practices for achieving responsible AI content generation.
What Are Generative AI Systems?
Generative AI refers to artificial intelligence capable of producing content, such as text, images, music, or video, based on patterns learned from vast datasets. These systems can compose emails, generate marketing campaigns, or develop code, requiring little to no manual intervention. While the creativity and efficiency of generative AI are undeniable, its growing influence introduces responsibilities for developers, content creators, and tech ethicists to ensure high-quality, reliable, and ethical content.
Content creation powered by generative AI has tremendous potential. However, with this potential comes the risk of misuse or unintended harm, particularly when outputs are unchecked. This is why controlling the output of generative AI systems is critical—not only for accuracy but also for upholding ethical standards across industries.
Key Reasons for Controlling AI Output
Preventing Misinformation
One of the most significant concerns surrounding generative AI is its potential to spread false or misleading information. These systems rely heavily on the data they have been trained on, and inaccuracies in the initial dataset can lead AI to generate statements that sound convincing but are factually incorrect.
For example, an AI-powered content generator might produce an article on health-related advice that conflicts with established medical standards. Without proper oversight, misinformation could quickly snowball, damaging public trust and credibility.
Actionable takeaway: Controlling AI-generated outputs ensures that content adheres to current, verified information standards, preventing the dissemination of harmful inaccuracies.
Avoiding Bias and Harm
AI models are only as good as the data they are trained on. If this data includes biases or prejudices, the system may inadvertently reproduce them in its outputs. This can result in discriminatory or harmful content that marginalizes specific groups, perpetuating societal inequities.
For instance, generative AI trained on historical recruitment data might favor male-dominated resumes when crafting job descriptions or evaluating candidate suitability due to implicit biases present in the training data.
Actionable takeaway: Monitoring outputs for bias ensures that generative AI remains inclusive, promoting fairness and equity.
User Safety and Privacy
Generative AI models often process sensitive user data, raising concerns about user privacy and trust. Exposing this information within generated content, whether intentionally or accidentally, is a major breach of privacy.
For example, AI systems might inadvertently integrate a user’s private information, such as personal email addresses or contact details, into generated communication or reports.
Actionable takeaway: By employing robust protections, businesses can mitigate risks to user privacy and promote transparency, safeguarding consumer trust.
Compliance and Regulation
AI-generated content must adhere to industry standards and legal requirements. For fields like finance, healthcare, and government, non-compliance could result in hefty fines, reputational damage, or even harm to human health and safety.
For instance, an AI system generating financial reports must adhere to compliance regulations for factual accuracy and data privacy while avoiding speculative or sensitive language.
Actionable takeaway: Governing compliance within AI systems’ generated outputs not only avoids legal complications but also ensures credibility and professionalism.
Challenges in Controlling AI Output
Complexity of AI Algorithms
AI models, though incredibly advanced, are inherently complex and often operate as “black boxes,” producing outputs that are difficult to trace back to specific input data or decision-making paths. This unpredictability complicates control efforts.
Balancing Creative Freedom with Responsibility
Generative AI thrives on creativity, but how do we define boundaries without diminishing its creative potential? Over-moderating an AI model might limit its ability to produce innovative solutions, while under-moderating could raise accuracy or ethical concerns.
Solutions and Best Practices
Implementing Strong Moderation and Filtering
Tools and strategies for moderating AI outputs can help ensure that harmful, biased, or inaccurate content is flagged and addressed. Developers and content creators should integrate filtering systems that catch errors, identify bias, and ensure adherence to quality standards.
- Example Tools: Automated content moderation platforms and bias-detection algorithms like Perspective API or Fairness Indicators.
Regular Updates and Monitoring
Because data constantly evolves, AI models must be periodically retrained and updated to reflect changes in societal norms, legal frameworks, and industry standards. Continuous monitoring ensures that no outputs stray from acceptable parameters.
- Example Practice: Conducting periodic audits on generated outputs and incorporating user feedback to enhance the system.
Transparent Development and Usage
AI developers and users should cultivate transparency by documenting how models are trained, what data sources were utilized, and what measures are in place to prevent misuse. Clearly communicating the system’s limitations to users promotes responsible adoption.
- Example Initiative: Publishing guidelines or disclaimers about the scope and boundaries of AI-generated outputs.
The Road Ahead for Generative AI
Controlling the output of generative AI systems is essential to ensuring accuracy, fairness, and user trust. Although challenges like algorithm complexity and balancing creative freedom persist, employing best practices like strong moderation, consistent updates, and transparent communication can mitigate risks.
As generative AI continues to evolve, the role of developers, content creators, and tech ethicists becomes increasingly vital. Together, we hold the power to shape systems that not only generate extraordinary content but also uphold the ethical standards and trust necessary in our technological landscape.
For organizations and individuals ready to harness AI responsibly, the path to better systems starts with implementing these solutions today.